Goal
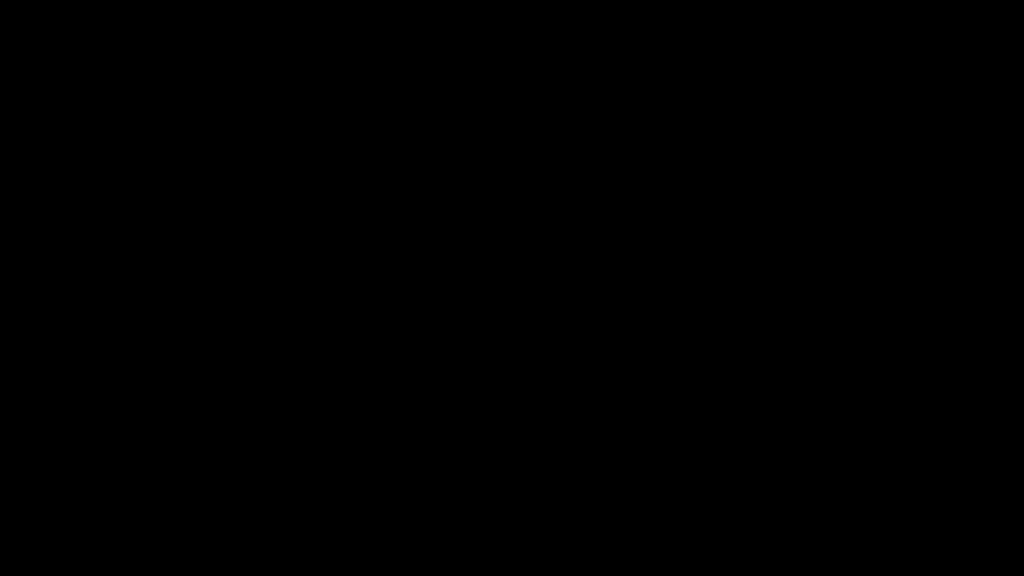
GIF courtey of EarthLoc&Match.
Astronaut Photography Localization (APL) is the task of identifying the location on Earth depicted in an image taken by astronauts in space with handheld cameras. In the microgravity environment, the astronauts can orient the cameras to image any portion of the visible Earth area (20M sqkm). When using high zoom lenses, the images may contain only a few sqkm of land area, making localization akin to finding a needle in a haystack.